Recently, Rui et al. published the journal pre-proof of their review “Machine learning in predicting antimicrobial resistance: a systematic review and meta-analysis” which will be published in the International Journal of Antimicrobial Agents. In their publication they used a substantial amount of literature to review and summarize the most used Machine Learning (ML) algorithms and methods to predict antimicrobial resistance (AMR), also measuring the quality of all analysed studies.
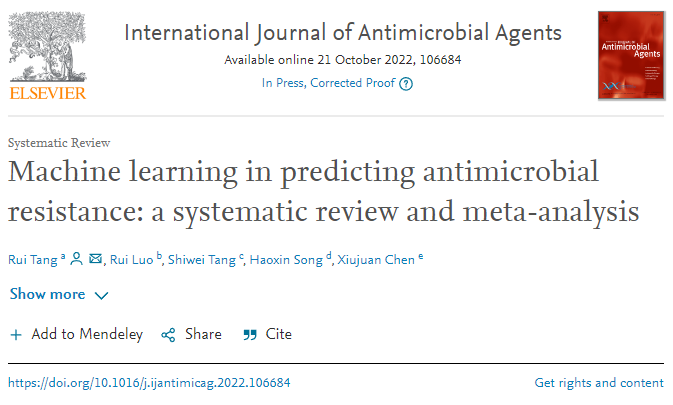
First of all, let’s dive into their findings about the most common ML algorithms. As their review follows, Linear Regression and Decision Tree were the most popular ML algorithms for AMR in the analysed studies. Those algorithms were followed by Random Forest. Other used ML algorithms were Support Vector Machine, k-Nearest Neighbors, Neural Networks and so on. Also, the metrics used for the evaluation of the different ML algorithms’ performances were inconsistent but the AUC score (Area Under the Curve), the Sensitivity, the Specificity, the PPV (Positive Predictive Value) and the NPV (Negative Predictive Value) are regularly used parameters. Finally, the most common method of validation was k-fold cross validation followed by leave-one-out cross validation. CLOVER MS Data Analysis Software (CLOVER MSDAS) has implemented all these features and metrics as well as the optimization of them for Mass Spectrometry (MS) and Infrared Spectroscopy (IR) data.
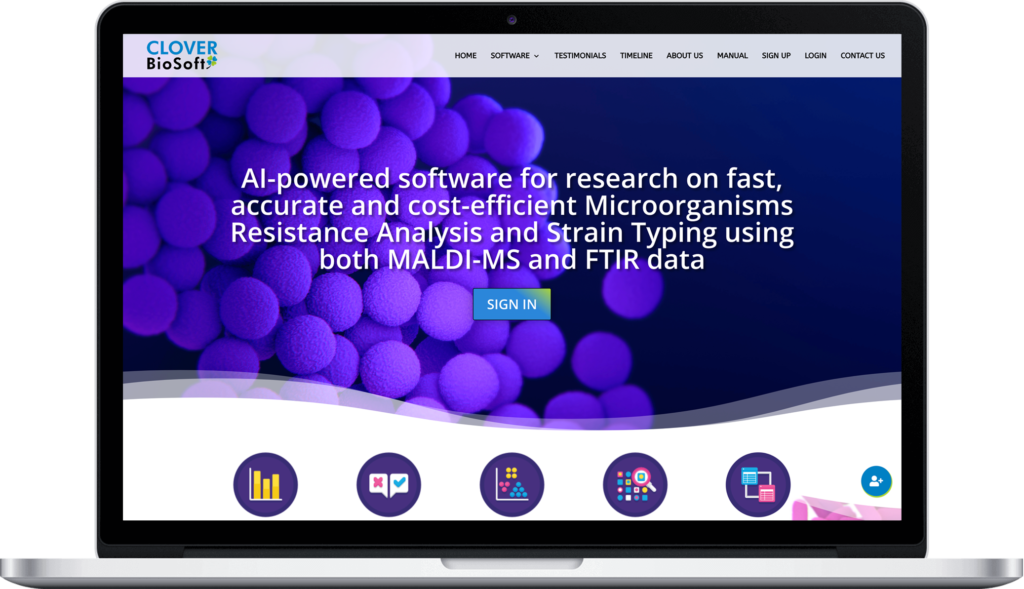
This review also made use of PROBAST, a tool to assess risk of bias and applicability of prediction model studies, for bias prediction based on medical information. From all 25 studies analysed, only one was rated with low risk of bias and another one was considered high risk for predictors’ applicability.
Lastly, as a final note on the reviewed article, in the studies analysed the variables used to predict AMR were diverse but in general, the most common inputs were demographic characteristics, factors causing resistant bacterial colonization or infection, previous resistant bacterial colonization or infection, indwelling catheter, prior antibiotic exposure, and microbiological information. Laboratory test values (blood cell counts, C-reactive protein…) also were used as predictors.
The published review concluded that ML could achieve satisfactory results in most of the reviewed studies. In fact, the review points out that ML prediction could be a potential technology for assisting in antibiotic selection.
Here in Clover Bioanalytical Software, we carry out the analysis of MALDI-TOF and FTIR data of bacterial cultures from resistant and not resistant strains obtained directly from infected patients. CLOVER MSDAS methodology fits into the ones described in the cited article which has proven high predictive potential for AMR. In fact, as other proof of the efficiency of ML algorithms to predict AMR, we have uploaded our publications in collaboration with different hospitals and research groups of reference on our website available to everyone interested in the subject.
CLOVER MSDAS has been developed to be a useful and intuitive platform where any researcher or technician can perform biological analysis such as Biomarker Analysis or use different classification algorithms as Random Forest Classification, k-Nearest Neighbors Classification, LightGBM among many others as well as validate the prediction models created. All these tools are available to predict AMR in a short period of time for the user.
In Clover Biosoft we perform high quality biostatistical analyses to develop innovative and efficient bioinformatic software. This software is developed by a highly motivated and hard-working interdisciplinary team and is already available to any professional, research group or institution. So, if you are interested in our work, we encourage you to contact us.